Unlocking Business Potential with Labeled Image Datasets
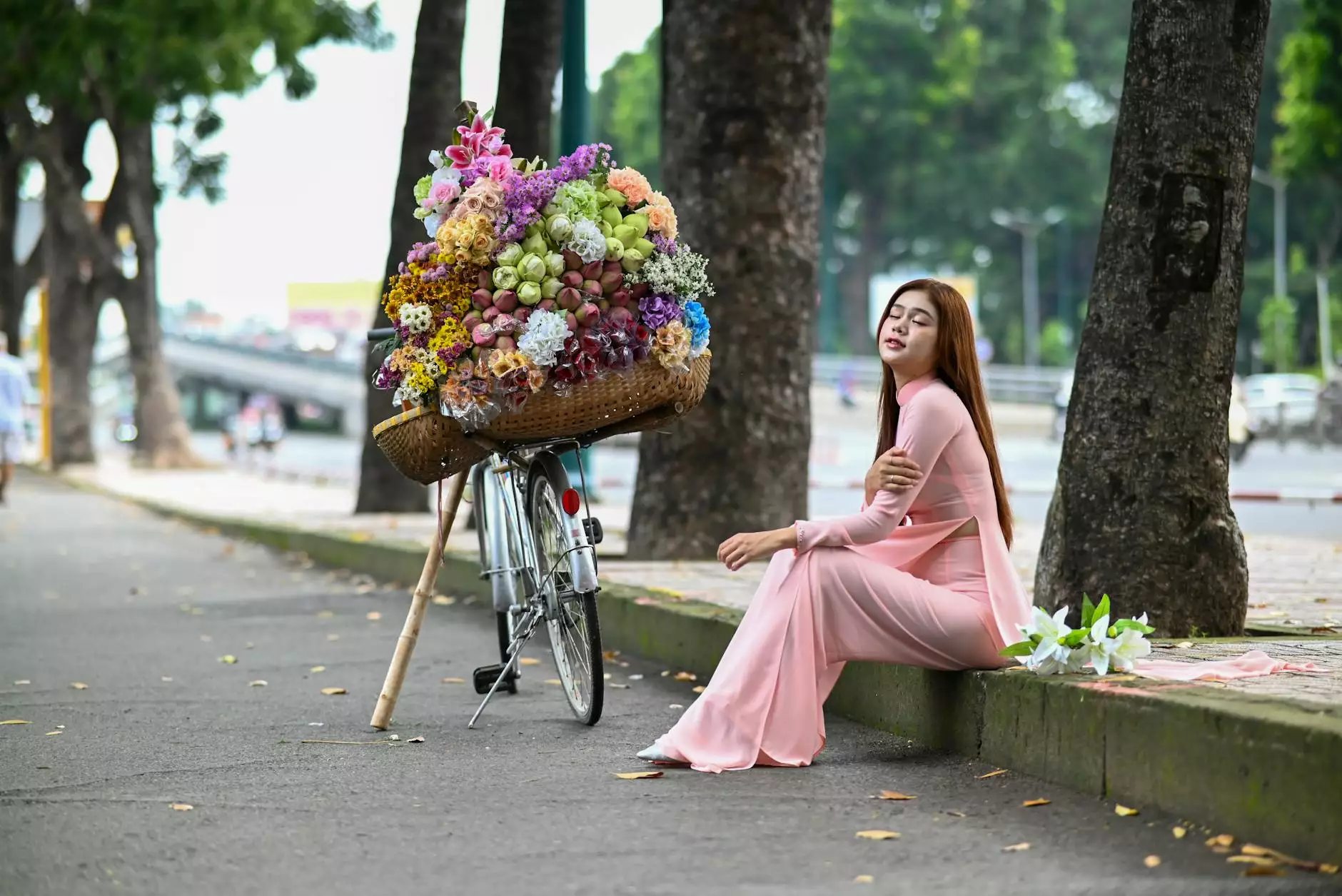
The Importance of Data Annotation in Modern Businesses
In today's rapidly evolving technological landscape, the need for accurate and efficient data handling is more critical than ever. Businesses are increasingly relying on labeled image datasets to train machine learning algorithms and artificial intelligence applications. The significance of data annotation cannot be overstated; it is the backbone of any data-driven strategy.
Understanding Labeled Image Datasets
A labeled image dataset consists of images that have been tagged or annotated with metadata, providing context for artificial intelligence (AI) models to understand various features within the images. This process is essential for training AI systems for tasks such as:
- Object Recognition: Identifying and classifying objects within images.
- Image Segmentation: Dividing an image into meaningful segments for more detailed analysis.
- Facial Recognition: Training systems to detect and recognize human faces.
- Scene Understanding: Enabling AI to understand the context of an image.
The Role of Data Annotation Tools and Platforms
Data annotation tools and platforms, such as those offered by keylabs.ai, play a pivotal role in creating and managing labeled image datasets. These platforms provide users with the necessary tools to annotate vast amounts of data quickly and efficiently, ensuring high-quality datasets that drive business success.
Key Features of an Effective Data Annotation Tool
When selecting a data annotation tool, businesses should consider several key features that enhance efficiency and effectiveness:
- User-Friendly Interface: A simple, intuitive interface is essential for maximizing productivity, allowing teams to focus on annotation rather than navigation.
- Collaboration Features: Tools that allow multiple users to work simultaneously can significantly speed up the annotation process.
- Integration Capabilities: The tool should easily integrate with existing systems and workflows to streamline operations.
- Quality Control Mechanisms: Built-in quality assurance processes help maintain the integrity of labeled image datasets.
- Scalability: As data demands grow, the tool must be able to scale, supporting larger projects without sacrificing performance.
Benefits of Using Labeled Image Datasets
The incorporation of labeled image datasets into business strategies offers numerous benefits:
- Enhanced AI and ML Models: Labeled data is critical for training machine learning models with high accuracy, enabling superior performance in tasks like image classification.
- Streamlined Workflows: Automating the annotation process reduces manual labor, allowing teams to focus on analysis and decision-making.
- Improved Accuracy: High-quality datasets minimize the risk of errors and biases that may occur with poor annotation practices.
- Better Customer Experience: Companies using AI-powered insights derived from accurately labeled datasets can better understand and meet customer needs.
Real-World Applications of Labeled Image Datasets
Various industries are leveraging labeled image datasets for a multitude of applications:
- Healthcare: In medical imaging, labeled datasets are crucial for developing diagnostic algorithms that can identify diseases from scans.
- Retail: AI systems driven by labeled image datasets are utilized for inventory management, customer interaction analysis, and targeted marketing strategies.
- Automotive: Self-driving car technologies rely heavily on labeled datasets to ensure accurate environmental perception and navigation.
- Security: Facial recognition systems depend on large, well-annotated datasets to function effectively in surveillance applications.
Challenges in Creating Labeled Image Datasets
While the benefits of labeled image datasets are significant, companies also face challenges in their creation and management:
- Time Consumption:
- Annotating images can be a labor-intensive process, especially for large datasets.
- Quality Assurance:
- Ensuring that annotations are accurate and consistent across the dataset can be difficult.
- Scalability Issues:
- As data requirements increase, maintaining efficiency in annotation becomes challenging.
Optimizing Your Data Annotation Process
To maximize the benefits of labeled image datasets, businesses should adopt several best practices in their annotation processes:
- Define Clear Guidelines: Establishing comprehensive annotation guidelines helps maintain consistency and clarity throughout the team.
- Leverage Automation: Utilizing AI-assisted annotation tools can significantly speed up the process while maintaining high quality.
- Invest in Training: Providing training for annotators ensures that everyone understands the processes and expectations, improving overall quality.
- Regularly Review Data: Implement a system for periodically auditing and verifying annotations to ensure ongoing accuracy.
Conclusion: Elevating Business Intelligence with Labeled Image Datasets
The ability to harness the potential of labeled image datasets can transform a business's approach to data. By investing in robust data annotation tools and platforms, such as those provided by keylabs.ai, organizations can streamline their data workflows, enhance their machine learning models, and ultimately drive greater success across their operations.
As businesses move into an increasingly data-driven future, the strategic use of labeled image datasets will be a key differentiator that separates leaders from laggards. Whether in healthcare, retail, or any other industry, the insights gained through effective data annotation are invaluable. Start harnessing the power of labeled image datasets today to unlock the next phase of your business growth.